Keynote Speakers
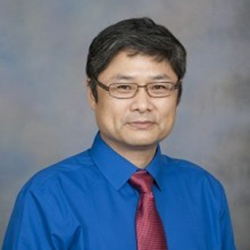
Peihua Qiu
Peihua Qiu is a professor and the founding chair of the Department of Biostatistics at the University of Florida. Qiu has made substantial contributions in the research areas of jump regression analysis, image processing, statistical process control, survival analysis, dynamic disease screening, and spatio-temporal disease surveillance.
So far, he has published two research monographs and over 140 research papers in refereed journals in these areas. He is an elected fellow of the American Statistical Association, an elected fellow of the Institute of Mathematical Statistics, an elected fellow of the American Society for Quality, and an elected member of the International Statistical Institute. He served as an associate editor for a number of top statistical journals, including the Journal of the American Statistical Association, Biometrics, and Technometrics. He was the editor of the flagship statistical journal Technometrics during 2014-2016.
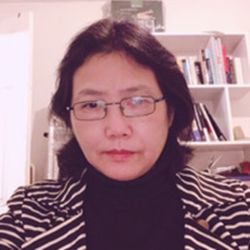
Dr. Hongmei (Mary) He
The trustworthiness of robots and autonomous systems (RAS) is at the centre of many research agendas on AI driven autonomous systems. This research systematically investigates for the first time the key aspects of human-centred AI (HAI) for trustworthy RAS in terms of safety, security, human-machine interaction, system health and ethics by identifying the challenges in implementing trustworthy autonomous systems with respect to the five key facets and exploring the role of AI in relation to the five facets of trustworthy RAS. It also presents a new acceptance model for RAS as a framework for human-centred AI requirements, promoting machine intelligence that augments human capabilities and places humans at the centre to achieve trustworthy RAS by design.
Discover the work of Dr. Hongmei (Mary) He, a Professor specializing in Future Robotics, Engineering, and Transport Systems at the University of Salford. With advanced degrees from Loughborough University, UK, and a background as a senior embedded system engineer at Motorola, China, Dr. Hongmei is at the forefront of AI research. Her expertise spans Cognitive Robotics, Cyber Security, Data/Sensor Fusion, and the safety of autonomous systems. An accomplished researcher, she has led numerous projects funded by top-tier institutions and has an extensive publication record. Dr. Hongmei also plays a significant role in the academic and professional community through her involvement with IEEE, the EPSRC peer-review college, and as a reviewer for the EU H2020 ICT Robotics Programme.
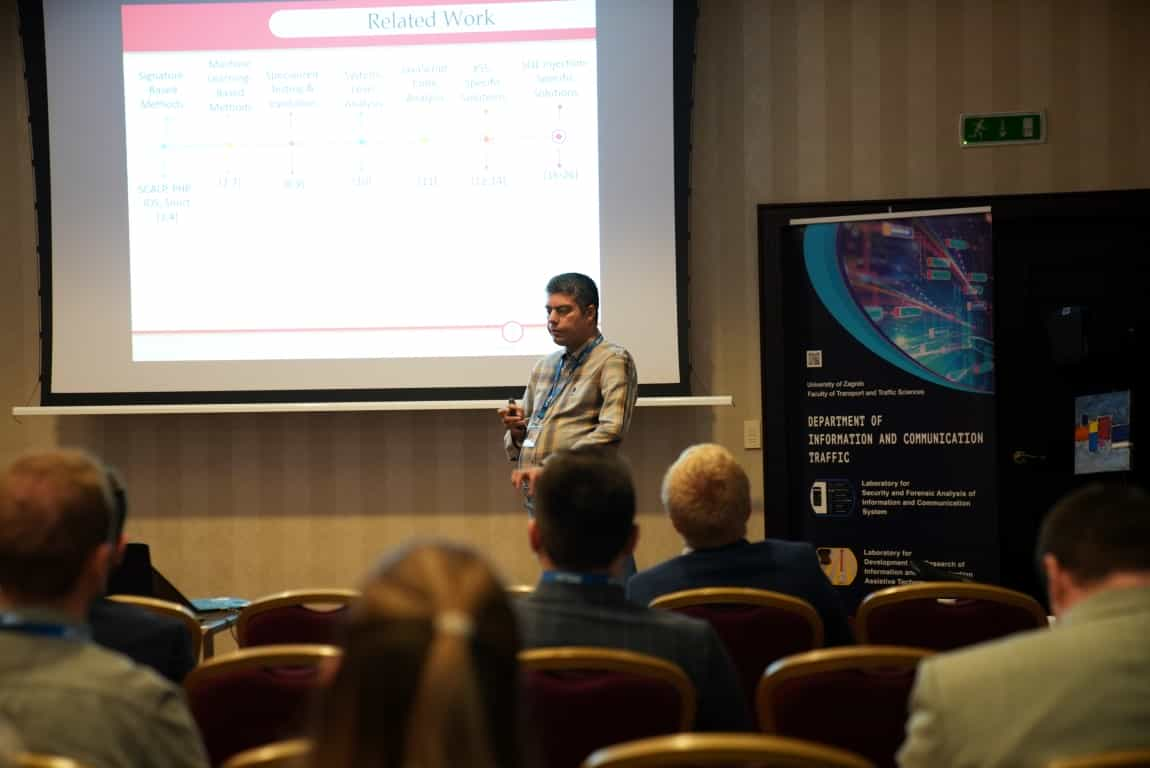
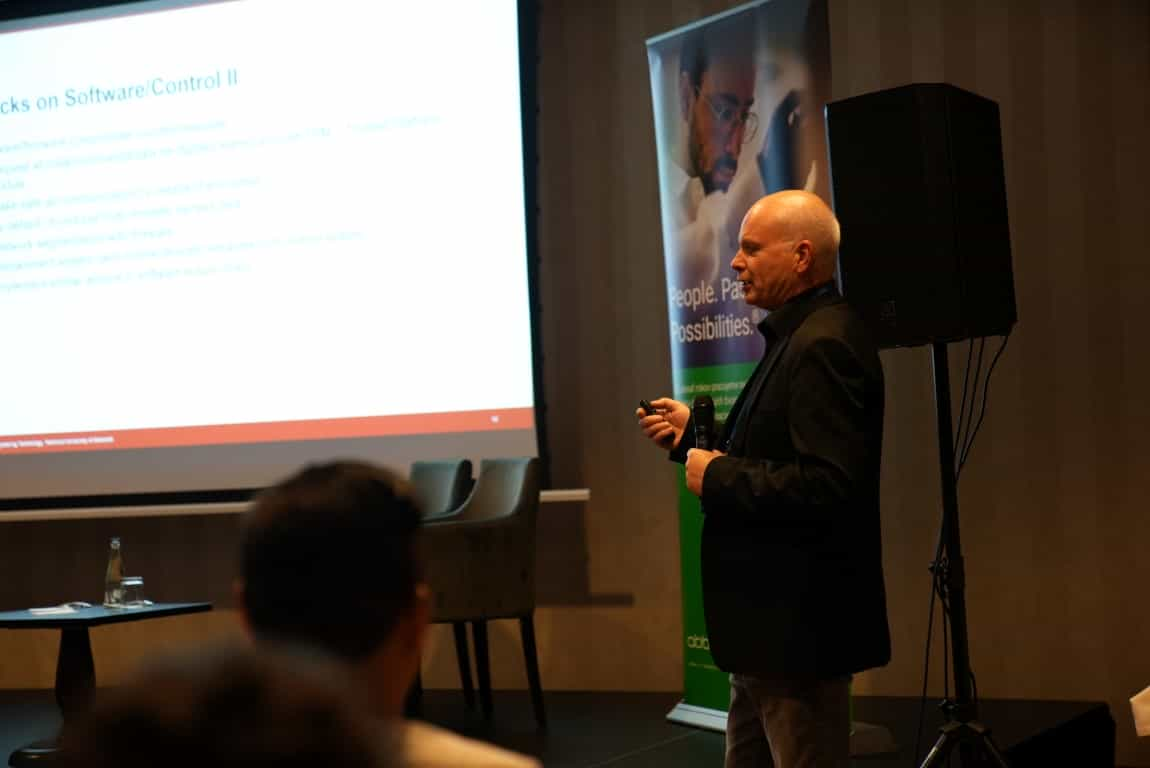
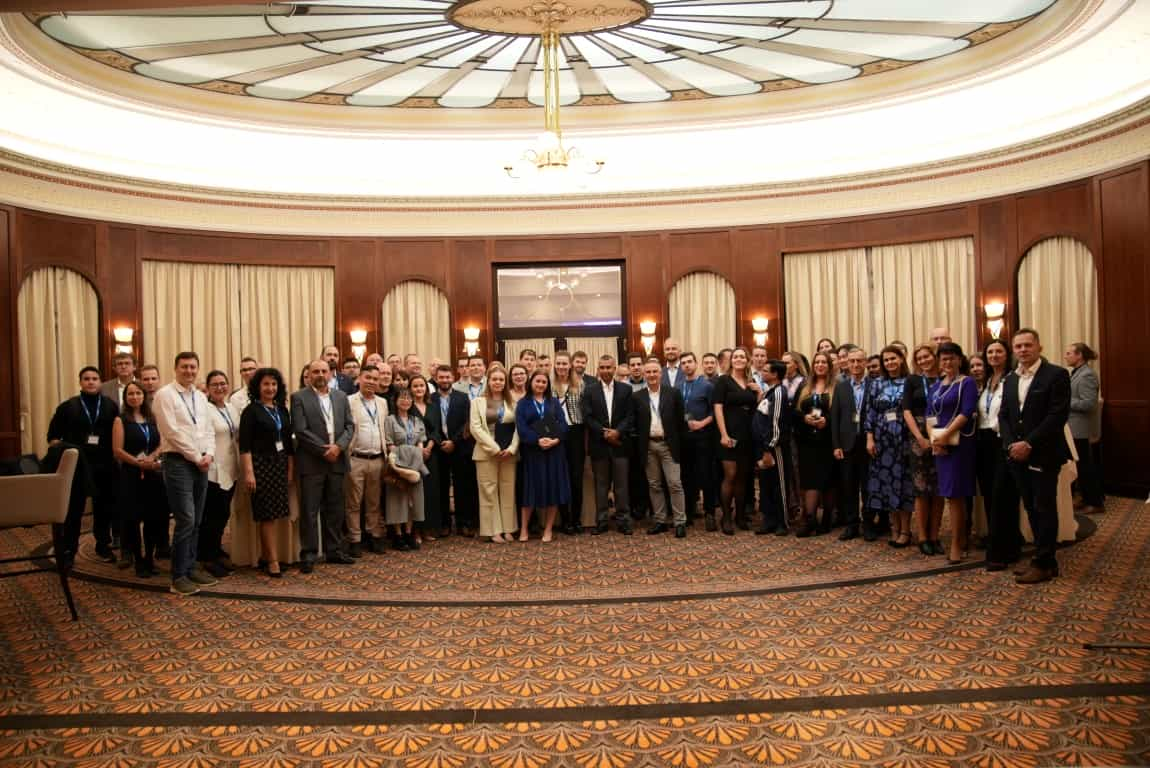
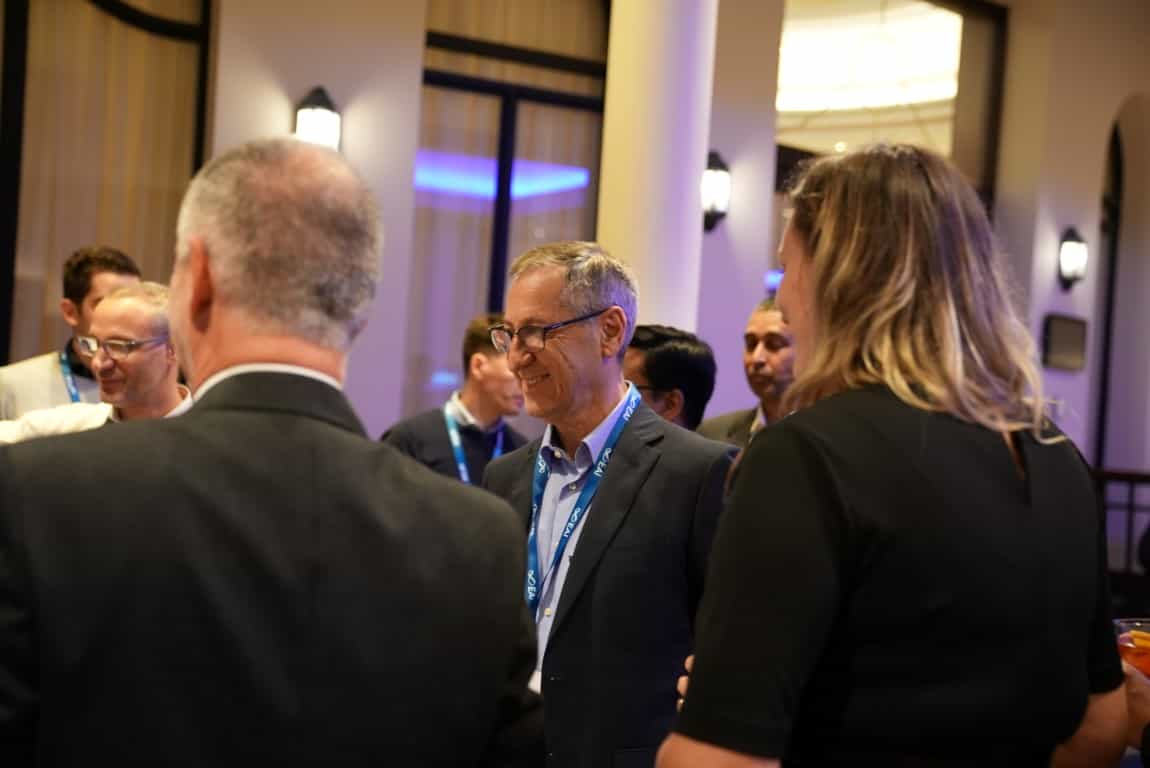
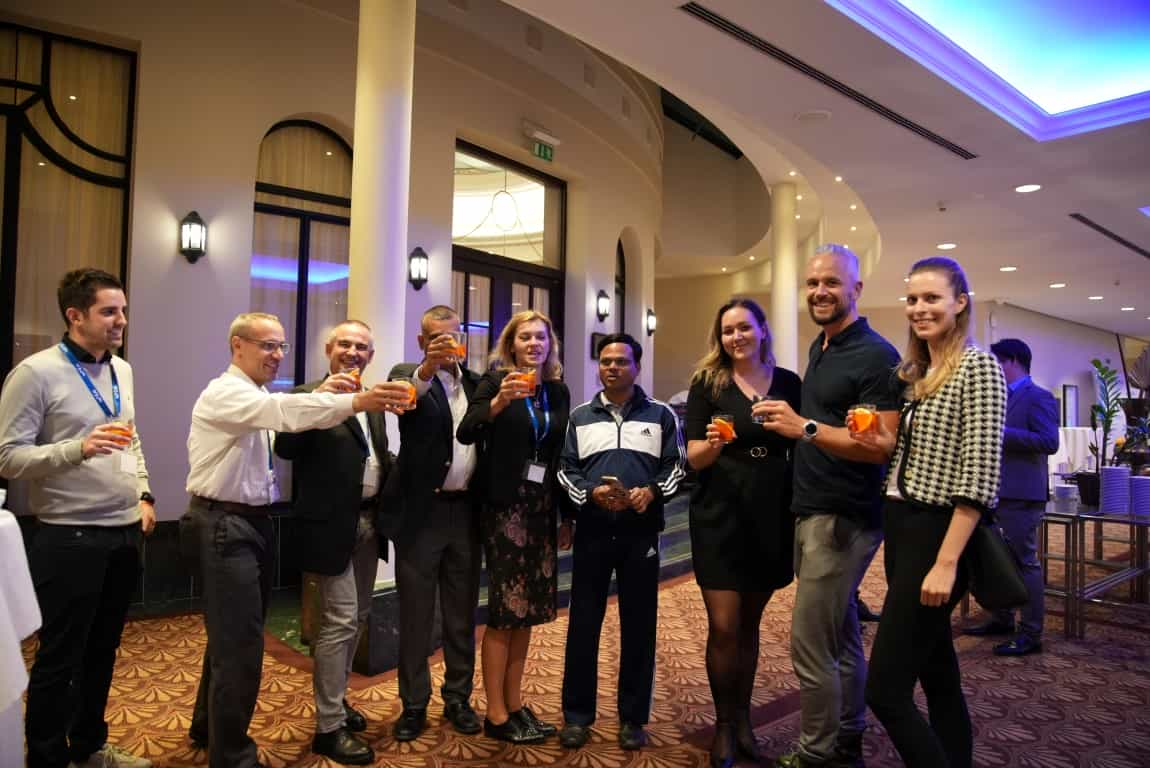
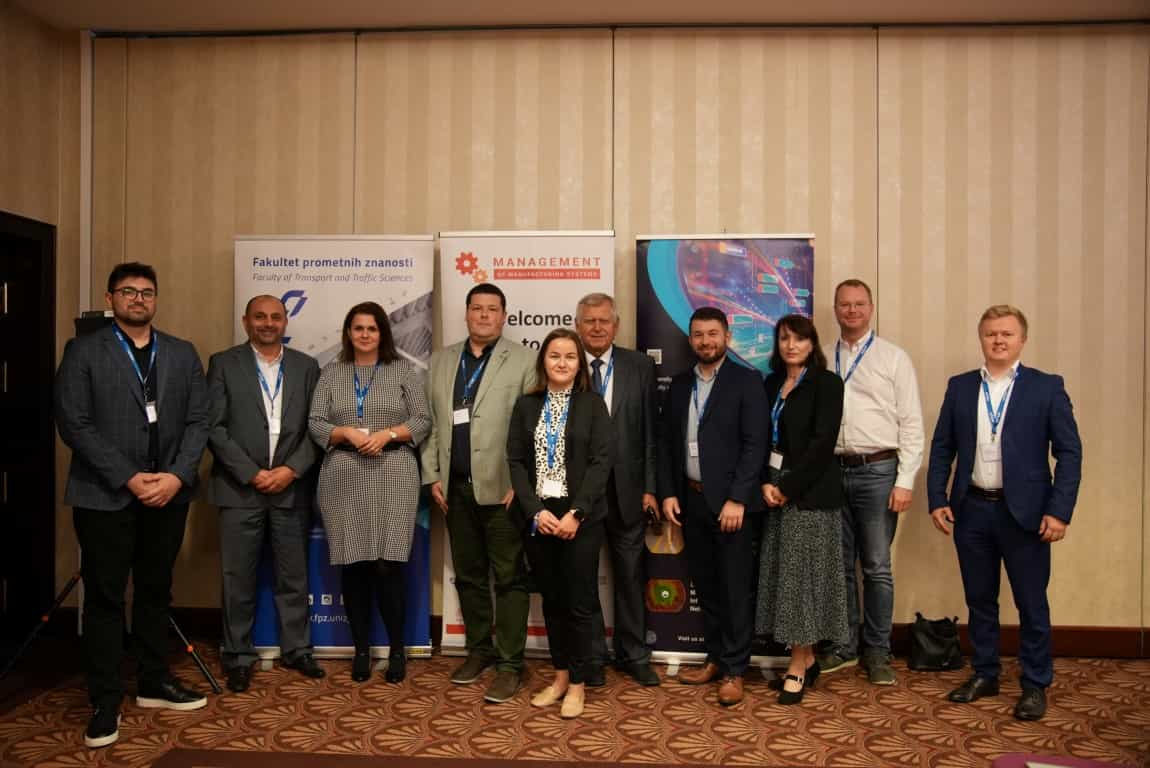
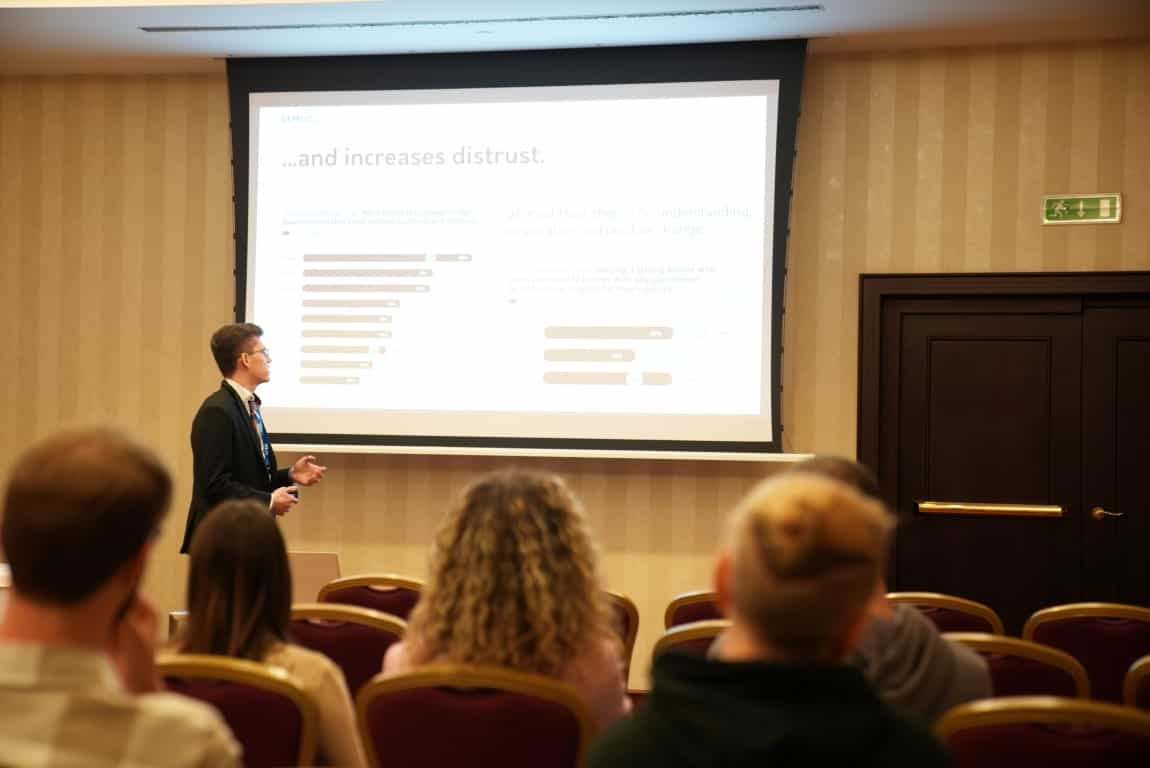
Flashback to Smart Life Summit 2023!
Relive the moments of innovation and inspiration from Smart Life Summit 2023 through our captivating photo gallery. Explore snapshots of insightful discussions, groundbreaking presentations, and vibrant networking sessions that defined this unforgettable event. Get a glimpse into the future of technology and lifestyle as we reflect on the highlights of this remarkable gathering. Join us on this nostalgic journey as we celebrate the spirit of innovation and collaboration showcased at Smart Life Summit 2023.